In your exploration of electrical machine design, you'll find that using surrogate models for multi-objective optimization offers new prospects and hurdles. These models simplify complex systems, allowing for better performance predictions while balancing efficiency and cost. Techniques like genetic algorithms enhance the design process, but managing design complexity and regulatory compliance remains challenging. As you look deeper into the subject, you'll discover innovative approaches and strategies that could transform how electrical machines are designed.
Key Takeaways
- Surrogate models reduce computational costs in electrical machine design, enabling efficient performance predictions and optimization.
- Multi-objective optimization techniques, like genetic algorithms, effectively balance conflicting design goals such as efficiency and cost.
- Challenges include managing design complexity, thermal management, and regulatory compliance within electrical machine design processes.
- Future directions emphasize the integration of surrogate-assisted design methods to enhance innovation and adaptability in the market.
- Continuous advancements in machine learning and statistical methods improve the predictive capabilities of surrogate models in multi-objective optimization.
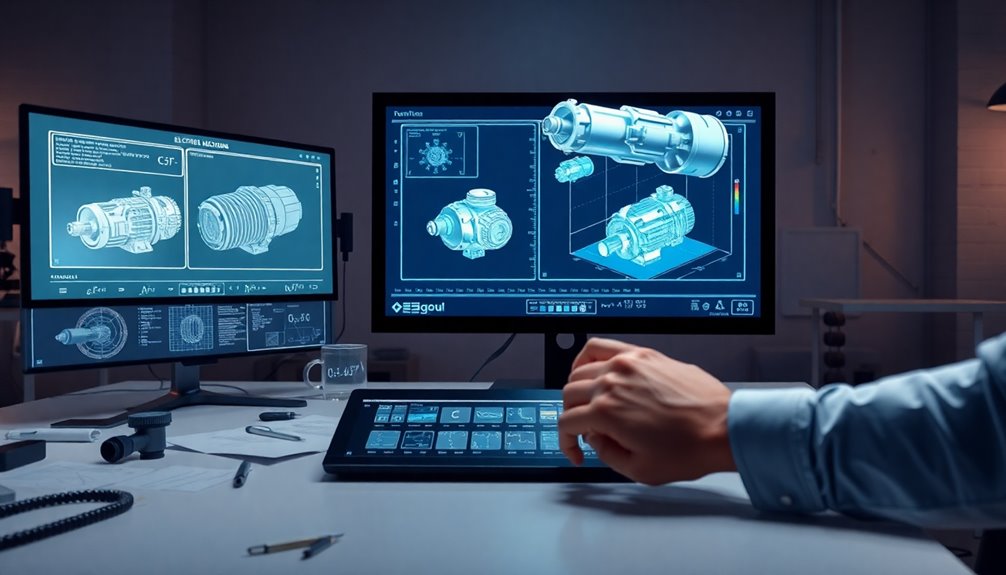
When you delve into electrical machine design, you'll quickly discover it's a complex field that requires balancing various factors like magnetic fields, efficiency, and cost. The design processes for different machine types, such as motors and generators, each present unique challenges. You'll often find yourself using finite element methods to simulate and optimize designs, which helps in visualizing performance before you even build a prototype. Books like "Electrical Machine Design" by V.S. Nagarajan can provide structured learning materials to guide you through this intricate landscape.
One of the most promising advancements in this field is the use of surrogate models for optimization. These models simplify complex systems, allowing you to predict performance without the computational burden typically associated with detailed simulations. Techniques such as gradient sampling and transfer learning enhance the optimization process, enabling you to tackle multiple objectives simultaneously. Additionally, the book offers objective questions that help reinforce your understanding of the material.
The emergence of surrogate models revolutionizes optimization, streamlining complex systems and enabling multi-objective performance predictions.
However, balancing conflicting goals, like efficiency and cost, remains a significant challenge. You'll want to explore various multi-objective optimization techniques, like genetic algorithms and Pareto optimization, as these can significantly improve your design outcomes.
Constructing effective surrogate models often involves statistical methods combined with machine learning, which can predict performance based on limited experimental data. This helps you refine designs for complex systems, such as five-phase permanent magnet synchronous machines, more efficiently.
Despite these advancements, challenges persist in electrical machine design. You'll have to manage design complexity, including magnetic field distributions and thermal management. Material selection is crucial too, as you need to find a balance between performance and cost.
Regulatory compliance adds another layer of complexity, as does the need for scalable solutions for large machines. In this competitive market, rapid technological advancements demand continuous innovation.
Embracing surrogate-assisted design with multi-objective optimization could well be the key to staying ahead, opening doors to future possibilities in electrical machine design.
Frequently Asked Questions
What Are Surrogate Models and How Do They Function in Optimization?
Surrogate models are tools that approximate complex objective functions, making optimization faster and more efficient.
You generate initial data points, then fit a model to this data, often using techniques like Gaussian Processes. These models enable quick evaluations, helping you explore the solution space effectively.
They aid in finding global optima by balancing exploration and exploitation, and can be combined with other optimization methods for enhanced performance.
How Does Multi-Objective Optimization Differ From Single-Objective Optimization?
Imagine navigating a bustling marketplace with countless stalls, each offering something unique.
That's multi-objective optimization for you! It differs from single-objective optimization, which is like choosing just one delicious dish.
In multi-objective scenarios, you're juggling multiple conflicting goals, seeking a balance. Instead of pinpointing a single solution, you find a spectrum of Pareto optimal solutions, each representing trade-offs.
It's about weighing options, embracing complexity, and making informed decisions that satisfy various needs.
What Industries Benefit the Most From Electrical Machine Design Optimization?
You'll find that the automotive, aerospace, industrial, and energy sectors benefit greatly from optimized electrical machine design.
In automotive, efficiency drives performance and meets emissions standards.
Aerospace gains from reduced weight and enhanced fuel efficiency.
Industrial applications see cost reductions and improved energy use.
Lastly, energy and home appliances leverage optimized designs to meet efficiency mandates and drive market growth.
Each sector thrives on innovation and sustainability, maximizing both performance and environmental impact.
Are There Specific Software Tools Recommended for Surrogate Modeling?
When you're diving into surrogate modeling, think of it like using a trusty Swiss Army knife.
You'll want to explore tools like Artificial Neural Networks for complex data, Gradient Boosting Decision Trees for torque behaviors, and the Finite Element Method for detailed simulations.
Don't forget cloud computing for scalable solutions and high-performance computing systems for fast execution.
Together, these tools will streamline your modeling process and enhance efficiency in your projects.
What Future Trends Are Expected in Electrical Machine Design Optimization?
Future trends in electrical machine design optimization will likely focus on integrating AI and machine learning for faster and more accurate design processes.
You'll see advancements in hybrid magnet configurations and sustainable materials, enhancing efficiency while reducing costs.
The rise of additive manufacturing will allow for complex geometries and improved thermal management systems.
Additionally, multi-objective optimization methods will evolve, enabling more precise balancing of performance parameters in innovative applications like electric vehicles and renewable energy systems.
Conclusion
In the quest for optimal electrical machine design, you might think surrogate models are your best friends, but they can be as fickle as a cat on a hot tin roof. While they promise efficiency and innovation, you'll find yourself navigating a maze of trade-offs and unforeseen challenges. Irony strikes as the very tools meant to simplify your work might complicate it further. So, embrace the chaos—they're just part of the journey toward that elusive perfect design.